Hierarchical Deep Reinforcement Learning for Legged Robot Navigation
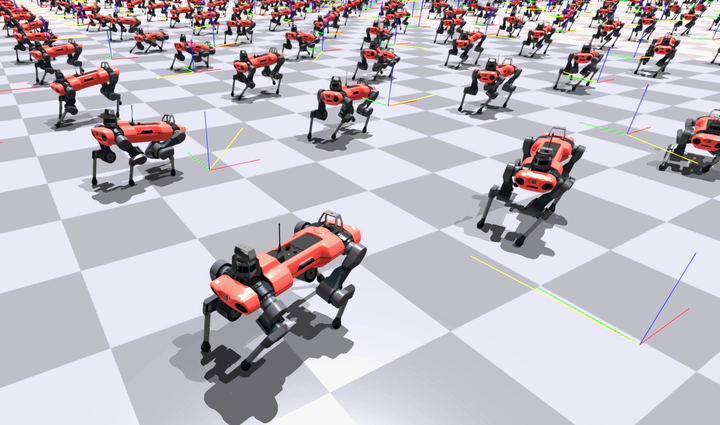
Description
High-level tasks such as pose tracking for legged systems are important yet challenging topics in mobile navigation. In scenarios where only high-level objectives are specified, obtained skills can be directly used as independent low-level components that break away from the high-level controller design. In this work, the effectiveness of an RL-based controller is proved with a proposed hierarchical control structure for a quadrupedal system where a high-level target-commanded policy learns to utilize existing low-level locomotion skills to continuously navigate the robot to track given pose trajectories on open and flat terrain. Experiments on a locomotion alignment task on ANYmal show that the functionality of the proposed RL-based controller is able to yield comparable tracking behaviors as a fine-tuned PD controller while providing additional safety guarantees and naturalness.