Gaussian Process Regression for Groundwater Pollution Prediction
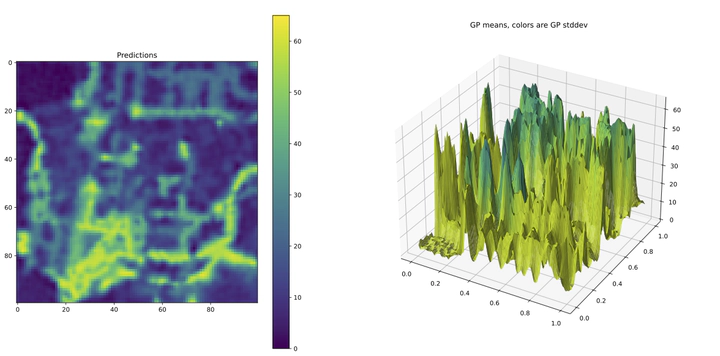
Description
The objective of the project is to predict groundwater pollution levels. To address the computational complexity associated with Gaussian Process models due to the large dataset, the Nyström approximation of the kernel function and inducing points method are applied. The kernel type and hyperparameters are tuned and learned during training. To handle the asymmetric cost, the prior mean is set to a non-trivial value. In this way, the possibility of underestimating the pollution value is reduced when there is large uncertainty about the prediction.